Sure,
Big Data Is Great. But So Is Intuition.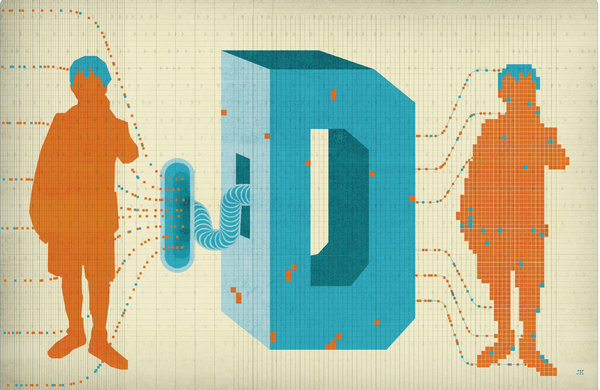
Published: December 29,
2012
It was the bold title of a conference this month at the Massachusetts Institute of Technology, and of a widely read article in The Harvard Business
Review last October: “Big Data:
The Management Revolution.”
Andrew McAfee, principal research
scientist at the M.I.T. Center
for Digital Business, led off the
conference by saying that Big Data would be “the next big chapter of our
business history.” Next on stage was Erik Brynjolfsson, a professor and director of the M.I.T. center and a co-author of the
article with Dr. McAfee. Big Data, said Professor Brynjolfsson, will “replace
ideas, paradigms, organizations and ways of thinking about the world.”
These drumroll claims rest on the premise that data like
Web-browsing trails, sensor signals, GPS tracking, and social network messages
will open the door to measuring and monitoring people and machines as never
before. And by setting clever computer algorithms loose on the data troves, you
can predict behavior of all kinds: shopping, dating and voting, for example.
The results, according to technologists and business
executives, will be a smarter world, with more efficient companies,
better-served consumers and superior decisions guided by data and analysis.
I’ve written about what is now
being called Big Data a fair bit
over the years, and I think it’s a powerful tool and an unstoppable trend. But
a year-end column, I thought, might be a time for reflection, questions and
qualms about this technology.
The quest to draw useful insights from business
measurements is nothing new. Big Data is a descendant of Frederick
Winslow Taylor’s “scientific management” of
more than a century ago. Taylor’s instrument of measurement was the stopwatch,
timing and monitoring a worker’s every movement. Taylor and his acolytes used
these time-and-motion studies to redesign work for maximum efficiency. The
excesses of this approach would become satirical grist for Charlie Chaplin’s
“Modern Times.” The enthusiasm for quantitative methods has waxed and waned
ever since.
Big Data proponents point to the Internet for examples of
triumphant data businesses, notably Google. But many of the Big Data techniques
of math modeling, predictive algorithms and artificial intelligence software
were first widely applied on Wall Street.
At the M.I.T. conference, a panel was asked to cite
examples of big failures in Big Data. No one could really think of any. Soon
after, though, Roberto
Rigobon could barely contain himself as he took to
the stage. Mr. Rigobon, a professor at M.I.T.’s Sloan School of Management,
said that the financial crisis certainly humbled the data hounds. “Hedge funds
failed all over the world,” he said.
The problem is that a math model, like a metaphor, is a
simplification. This type of modeling came out of the sciences, where the
behavior of particles in a fluid, for example, is predictable according to the
laws of physics.
In so many Big Data applications, a math model attaches a crisp number to
human behavior, interests and preferences. The peril of that approach[B1] , as in finance, was the subject of a recent book by Emanuel
Derman, a former quant at Goldman Sachs and now a
professor at Columbia University. Its title is “Models. Behaving. Badly.” Note by Robert Tischer (2012):
Big Data
Microsoft’s next generation of apps “will have the
ability to incorporate large amounts of new data obtained in real time” an
article in the New York Times reports. So what’s wrong with that? The problem
lies in the question of from where the data comes and when each piece arrives.
Today’s data paradigm is based on what the linguist Roy Harris calls the
Talking Heads model of linguistics. Big Data implies that these source
questions are irrelevant and immaterial and might as well be context-less
talking heads. Today’s unquestioned doctrine that data stored in databases and
clouds, separated from its intellectual moorings (i.e., context free data), I
submit, is flawed. At the heart of the discussion is whether asynchronous data,
that is, un-moored data stored in databases and servers, with their varying
(i.e., asynchronous) update latencies and intermittencies, is keeping us from
doing real distributed search and how synchronous search can remedy that.
Claudia
Perlich, chief scientist at Media6Degrees, an online
ad-targeting start-up in New York, puts the problem this way: “You can fool
yourself with data like you can’t with anything else. I fear a Big Data
bubble.”
The bubble that concerns Ms. Perlich is not so much a
surge of investment, with new companies forming and then failing in large
numbers. That’s capitalism, she says. She is worried about a rush of people
calling themselves “data scientists,” doing poor work and giving the field a
bad name.
Indeed, Big Data does seem to be facing a work-force
bottleneck.
“We can’t grow the skills fast enough,” says Ms. Perlich,
who formerly worked for I.B.M. Watson Labs and is an adjunct professor at the
Stern School of Business at New York University.
A report last year by the McKinsey Global Institute, the research arm of the consulting firm,
projected that the United States needed 140,000 to 190,000 more workers with
“deep analytical” expertise and 1.5 million more data-literate managers,
whether retrained or hired.
Thomas H. Davenport, a visiting
professor at the Harvard Business School, is writing a book called “Keeping Up
With the Quants” to help managers cope with the Big Data challenge. A major
part of managing Big Data projects, he says, is asking the right questions: How
do you define the problem? What data do you need? Where does it come from? What
are the assumptions behind the model that the data is fed into? How is the
model different from reality?
Society might be well served if the model makers pondered
the ethical dimensions of their work as well as studying the math, according to
Rachel Schutt, a senior statistician at Google Research.
“Models do not just predict, but they can make things
happen,” says Ms. Schutt, who taught a data science course this year at
Columbia. “That’s not discussed generally in our field.”
Models can create what data scientists call a behavioral
loop. A person feeds in data, which is collected by an algorithm that then
presents the user with choices, thus steering behavior.
Consider Facebook. You put personal data on your Facebook
page, and Facebook’s software tracks your clicks and your searches on the site.
Then, algorithms sift through that data to present you with “friend”
suggestions.
Understandably, the increasing use of software that
microscopically tracks and monitors online behavior has raised privacy worries.
Will Big Data usher in a digital surveillance state, mainly serving corporate
interests?
Personally, my bigger concern is that the algorithms that
are shaping my digital world are too simple-minded, rather than too smart. That
was a theme of a book by Eli Pariser, titled “The Filter Bubble: What the Internet Is Hiding From You.”
It’s encouraging that thoughtful data scientists like Ms.
Perlich and Ms. Schutt recognize the limits and shortcomings of the Big Data
technology that they are building. Listening to the data is important, they
say, but so is experience and intuition. After all, what is intuition at its
best but large amounts of data of all kinds filtered through a human brain
rather than a math model?
At the M.I.T. conference, Ms. Schutt was asked what makes
a good data scientist. Obviously, she replied, the requirements include
computer science and math skills, but you also want someone who has a deep,
wide-ranging curiosity, is innovative and is guided by experience as well as
data.
“I don’t worship the machine,” she said.
|